3-D Integrated In-Memory Deep Learning Hardware
As Moore’s law is showing signs of slowdown, a promising alternative to continue scaling the performance of computing platforms is to vertically stack multiple silicon dies into a heterogeneous 3-D (H3D) system. H3D integration technologies provide the flexibility to combine different process nodes and high-bandwidth data transmission.
Research Directions:
- Logic-on-memory and logic-on-logic 3-D integration architectures
- Back-end-of-line (BEOL) logic & memory devices and their applications
- Thermal, signaling, and power delivery management strategies in 3-D processors
One of our prior work, the H3DAtten accelerator, involves a 5-tier stack and marries the merits of multiple CIM paradigms through H3D integration to target the vision transformer workloads. For non-volatile on-chip storage of linear layer parameters, we use the RRAM cell for its compact cell size and low leakage power. For the intermediate matrix multiplications without trainable model parameters, volatile SRAM cache is a more suitable candidate for its high memory endurance and high access speed.
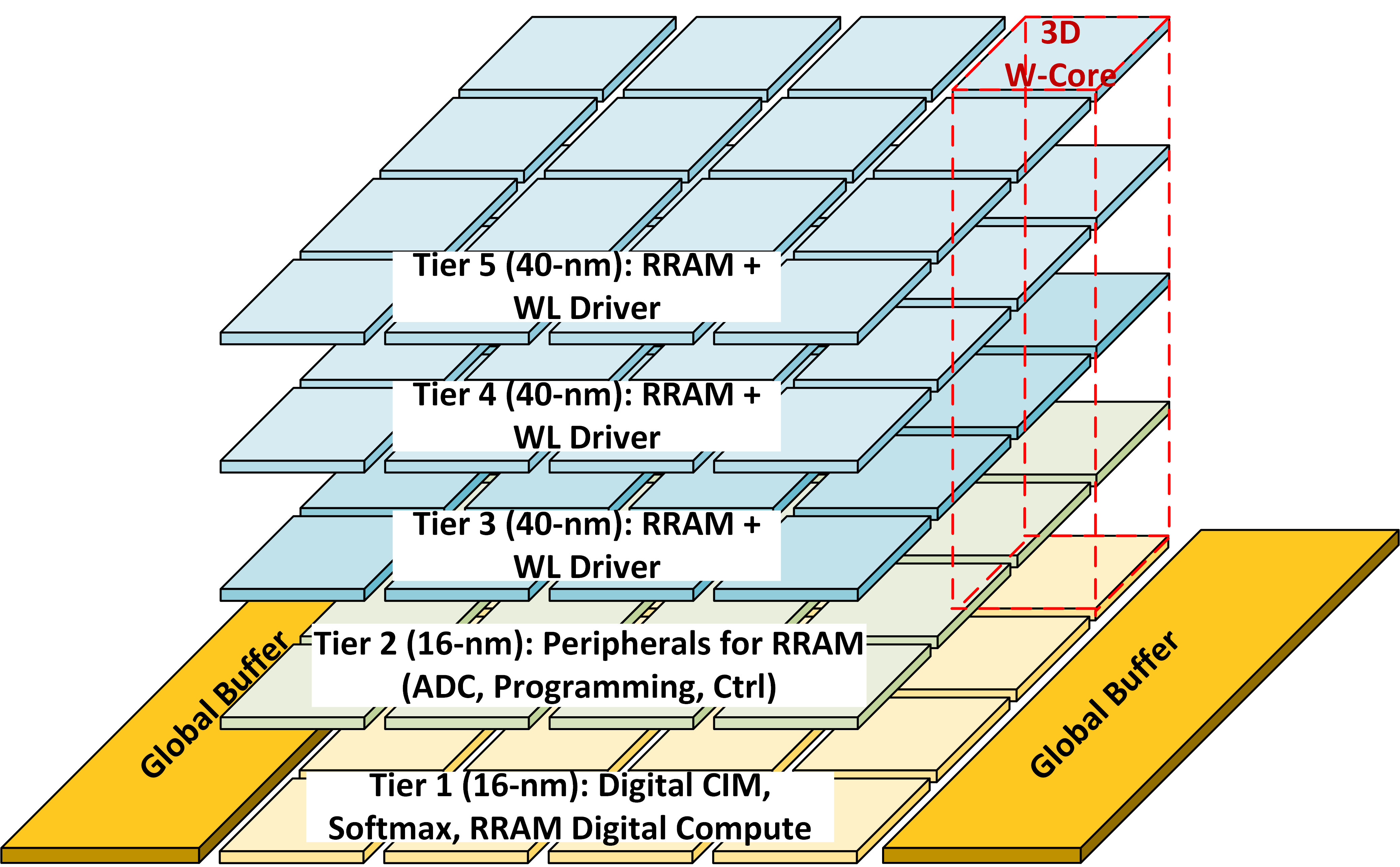
One critical aspect in designing a 3-D integrated hardware is its thermal profile, as stacking active dies can raise the junction temperature and leads to performance and reliability losses. We also model the entire 3-D stack to inspect potential heat dissipation issues.
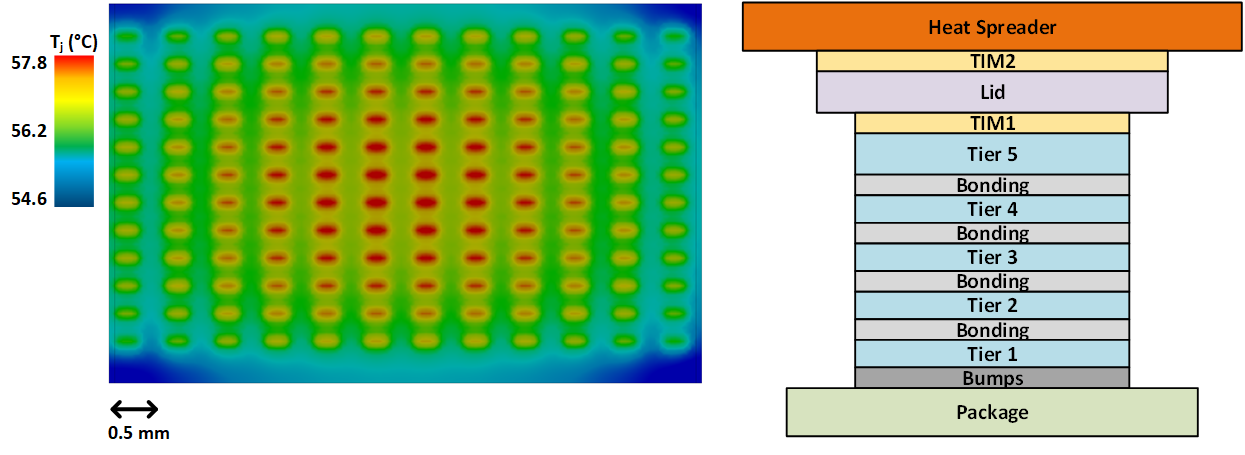