Low-Power Medical Electronics with On-Device Intelligence
By minimizing power consumption of battery-powered medical devices that perform monitoring, diagnostics, or intervention, they can function for extended periods without frequent recharging. Lightweight on-device intelligence enables real-time analysis and decision-making, which is critical in medical scenarios where immediate responses are necessary. Co-optimized algorithms and their hardware platform also allow for opportunities to directly process the medical data without reliance on host or cloud computing.
One line of research is power reduction techniques for diagnostic imaging, including portable ultrasound device. Low power consumption of portable ultrasound is especially critical for its application in remote and point-of-care clinical scenarios. Subsampling the ultrasound analog frontend channels is effective for reducing the system power consumption, and sparse signal reconstruction is subsequently needed to guarantee the final image quality for diagnosis. In this work, we aim to enable low-power power ultrasound imaging by approaching sparse reconstruction from both the algorithm and hardware fronts.
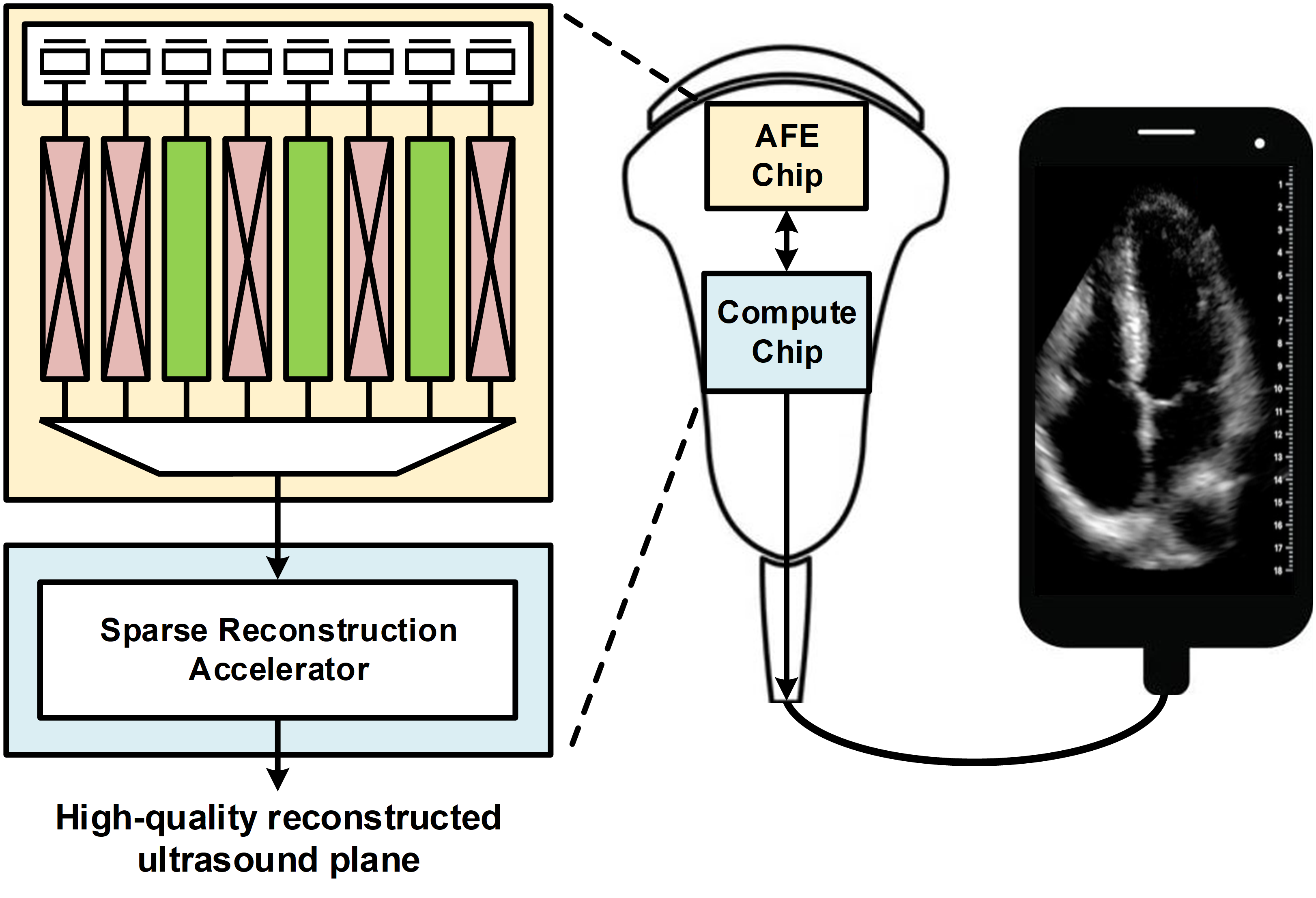
For sparse reconstruction, we design a compact neural network that allows at most 8× channel subsampling and still maintains high reconstruction quality. We also design a digital CIM accelerator to efficiently process the model near the ultrasound frontend. The proposed row-centric inter-layer pipelining addresses the challenge of accelerating U-Net models, significantly reducing the amount of required intermediate storage.
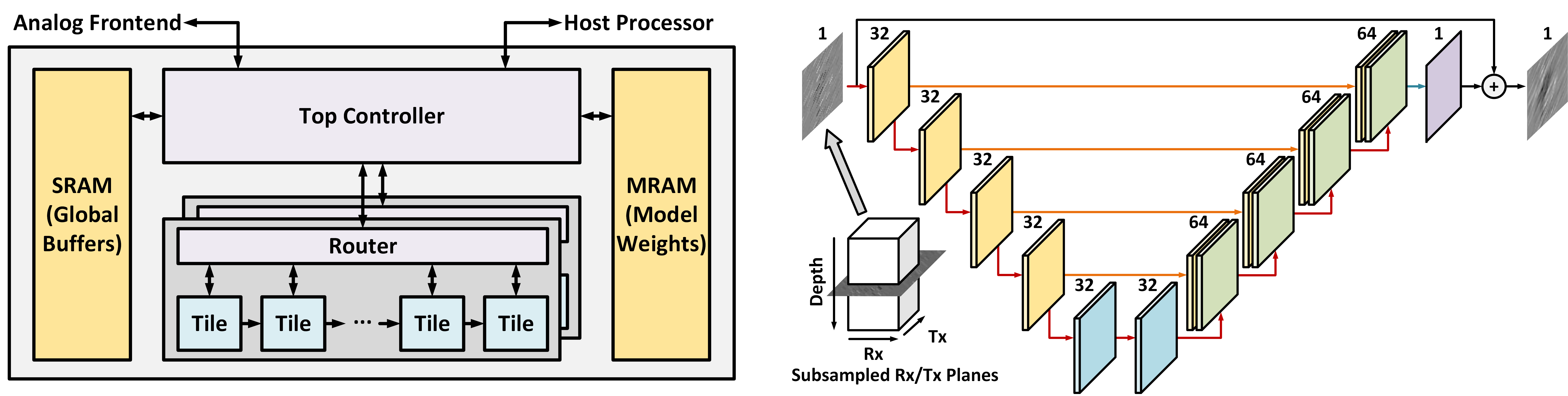
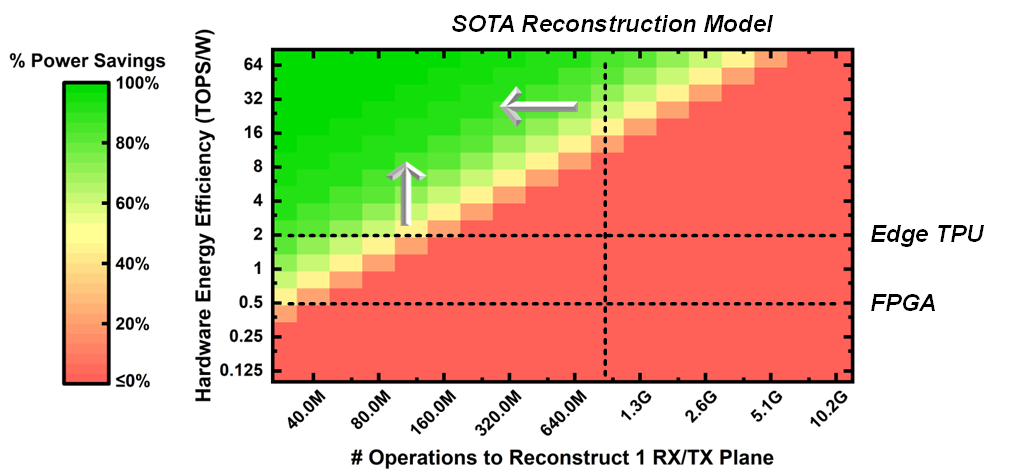